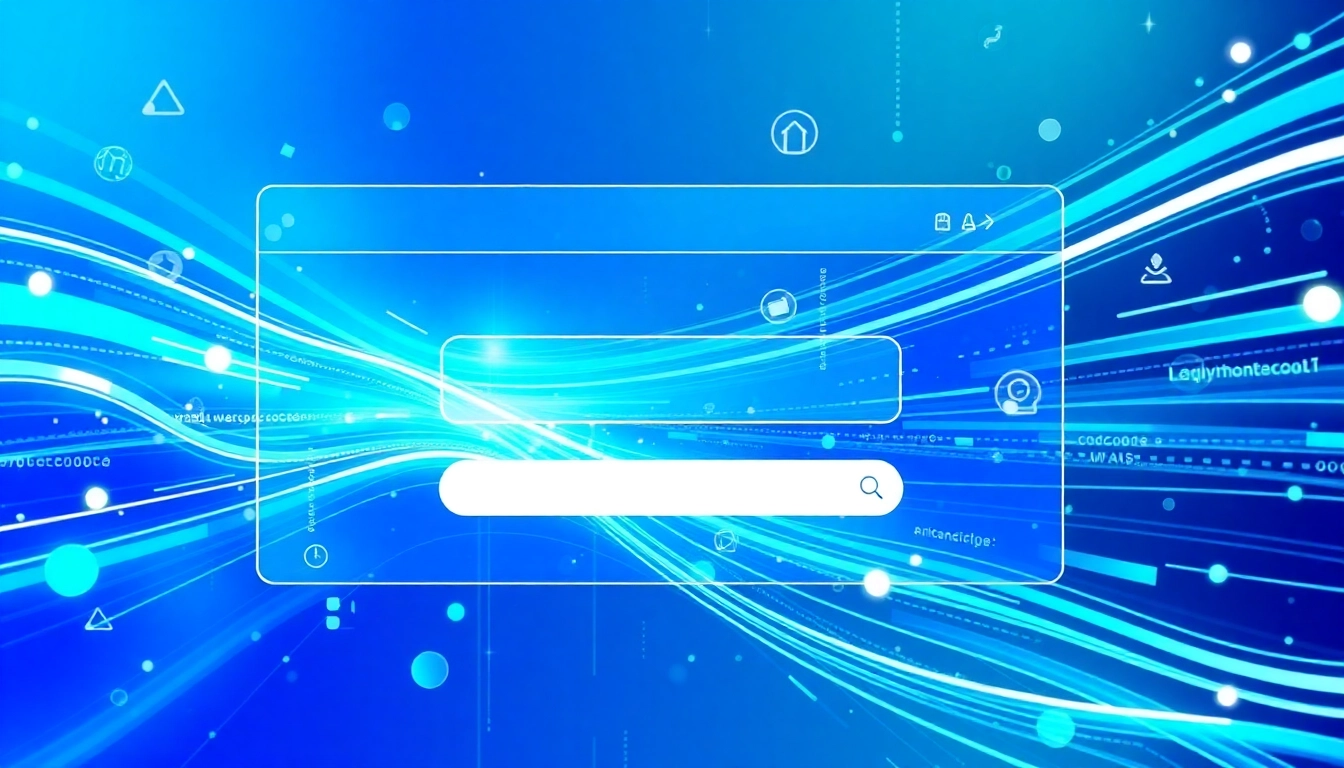
Introduction to AI Search
In an increasingly digital world, the significance of efficient and effective search technologies continues to grow. With the rapid evolution of artificial intelligence (AI), the way we retrieve information has undergone a revolutionary transformation. This new paradigm, referred to as AI search, leverages machine learning algorithms and natural language processing to provide users with more accurate, context-aware, and relevant search results than traditional search engines. Understanding the fundamental aspects of AI search, from its historical evolution to current trends, is essential for businesses and individuals alike as they navigate the digital landscape.
Defining AI Search and its Importance
AI search can be defined as the integration of artificial intelligence technologies into search engine algorithms to enhance the search experience. By analyzing vast amounts of data and understanding user intent, AI search engines can deliver personalized results, making them more user-centric than ever before. This advancement is crucial because as consumers become more accustomed to instant gratification and personalized experiences, the demand for sophisticated search solutions grows.
History and Evolution of Search Technologies
The evolution of search technologies can be traced back to the early days of the internet when simple keyword-based search algorithms dominated. These early systems relied heavily on indexing keywords without any consideration for context or user intent. As technology progressed, search engines began incorporating more complex algorithms, including Boolean logic, to improve search accuracy.
The introduction of AI into search technologies marks a significant shift, particularly with the arrival of machine learning and natural language processing in the 2010s. This integration allows search engines to better understand queries, discern context, and provide more nuanced results. Today’s AI search technologies utilize various methods, including deep learning and semantic search, to ensure users receive the most relevant information quickly.
Current Trends and Innovations in AI Search
Recent developments in AI search indicate a movement towards multimodal search capabilities, where users can input various forms of data such as text, image, and voice to retrieve relevant answers. Google’s Gemini, for example, incorporates AI chatbots to enhance the search process, allowing users to interact in a conversational manner. Moreover, the focus on improving transparency through citations in AI-generated content highlights the tech industry’s commitment to addressing ethical concerns in information retrieval.
Understanding AI Search Engines
What Makes AI Search Different?
The differentiation of AI search engines from traditional search engines lies in their ability to learn and adapt. Unlike conventional search engines that provide results based strictly on keyword matching, AI search engines leverage sophisticated algorithms that understand the context and intent behind queries. This artificial intelligence helps generate dynamic results and improves over time with feedback and interaction.
- Natural Language Processing (NLP): This technology allows users to search using natural language phrases rather than specific keywords, making search more intuitive.
- Contextual Understanding: AI search engines consider various factors such as location, user history, and device being used to tailor results.
- Continuous Learning: The ability to learn from user interactions enables AI search engines to refine their algorithms continually, adapting to changing user behaviors and preferences.
Key Players in the AI Search Market
Numerous companies are vying for dominance in the AI search space, each offering unique perspectives and technologies. Key players include:
- Google: With innovations like Gemini, Google leads the market by integrating AI-driven features into its search engine, enhancing both functionality and user experience.
- Microsoft: The integration of AI capabilities in Microsoft’s Bing with tools like Copilot transforms traditional search into a more interactive experience.
- OpenAI: The introduction of ChatGPT search illustrates OpenAI’s commitment to enhancing how search results are generated and presented, emphasizing nuanced and conversational interfaces.
Evaluating AI Search Engine Performance
Monitoring the performance of AI search engines is vital for understanding their effectiveness and impact. Several metrics can aid in evaluating their success:
- Precision and Recall: These metrics help assess the relevance of search results and their ability to retrieve all relevant documents.
- User Engagement: Analyzing click-through rates and time spent on pages can provide insights into user satisfaction.
- Feedback Mechanisms: Incorporating user feedback to improve algorithm accuracy is essential for continuous enhancement.
Implementing AI Search Solutions
Choosing the Right AI Search Platform
When selecting an AI search platform, organizations need to consider several key factors to ensure optimal performance and compatibility with existing systems:
- Scalability: The platform should be able to handle increasing amounts of data and user queries as the organization grows.
- Integration Capabilities: Ensuring that the AI search solution can seamlessly integrate with existing infrastructures and datasets is crucial for successful implementation.
- User Interface: A user-friendly interface can enhance user experience and increase adoption rates among employees.
Integrating AI Search into Existing Systems
Successfully implementing AI search requires an informed strategy to align the technology with existing systems:
- Conduct a Needs Assessment: Evaluate specific organizational needs and define clear objectives for the AI search implementation.
- Build a Roadmap: Create a detailed implementation plan outlining key milestones, responsibilities, and timelines.
- Training and Support: Provide adequate training for staff to ensure they are comfortable using the new system and encourage feedback during the transition.
Measuring Success and ROI of AI Search
To determine the effectiveness of an AI search implementation, organizations should establish clear KPIs (Key Performance Indicators) that align with their goals:
- User Satisfaction Surveys: Regular surveys can gauge user sentiment regarding the AI search functionality.
- Operational Efficiency: Analyze how AI search impacts business operations and workflow efficiencies.
- Return on Investment: Measure increased revenue or cost savings attributable to enhanced search capabilities.
Challenges and Limitations of AI Search
Common Pitfalls in AI Search Implementation
While AI search presents numerous advantages, organizations often face challenges during implementation. Common pitfalls include:
- Inadequate Data Quality: Poor data quality can lead to inaccurate or irrelevant search results.
- Lack of Clear Objectives: Without defined goals, AI search implementations can become unfocused and fail to deliver expected benefits.
- Underestimating Training Needs: Insufficient training for users can lead to low adoption rates and poor user experience.
Data Privacy and Ethical Considerations
As AI search technologies continue to evolve, important ethical and privacy concerns surrounding data handling must be prioritized:
- Data Security: Organizations must ensure robust data protection measures to prevent breaches and unauthorized access.
- User Consent: Transparency in how user data is utilized should be upheld to foster trust and comply with regulations.
- Bias Mitigation: Continuous evaluation of algorithms is essential to identify and reduce inherent biases that may arise in AI-generated results.
Future Challenges for AI Search Technologies
The landscape of AI search is ever-evolving, and future challenges may encompass:
- Continued Advancement in AI Capabilities: The pace of change may outstrip organizational adaptation, creating a gap between technology and usability.
- Keeping Up with Ethical Standards: Organizations will need to navigate the evolving expectations related to data privacy and ethical AI use.
- Managing User Expectations: As AI search capabilities become more advanced, users will expect increasingly sophisticated results, necessitating continuous improvements and updates in the technology.
The Future of AI Search
Predictions for AI Search Evolution
The future of AI search promises to be exciting, with several key predictions shaping the trajectory of the technology:
- Increased Personalization: AI will enable search engines to deliver hyper-personalized results based on nuanced user behavior and preferences.
- Integration of Multimodal Inputs: Users will increasingly interact with search engines through voice, images, and other inputs, requiring more sophisticated models for interpreting varied data types.
- Collaborative Search Environments: AI search tools are likely to be combined with collaborative platforms, allowing users to engage in joint data retrieval.
How to Stay Ahead in AI Search Innovation
Organizations that wish to maintain a competitive edge in AI search must adopt proactive strategies:
- Invest in Ongoing Research: Staying updated on the latest advancements in AI technologies can drive innovation and ensure relevance.
- Encourage Feedback and Adaptation: Continuously gathering user feedback facilitates necessary adaptations and improvements to AI search tools.
- Collaborate with Experts: Partnering with AI specialists and researchers can provide insights and help in navigating the complexities of advanced search technologies.
Conclusion: Embracing AI Search for a Smarter Future
The rise of AI search technologies marks a significant shift in how we interact with information. By embracing these advanced tools, organizations can unlock new levels of efficiency and user satisfaction. As AI continues to evolve, staying informed and adaptable will be crucial in tapping into the full potential of AI search, ensuring that both users and businesses can thrive in an increasingly complex information landscape.