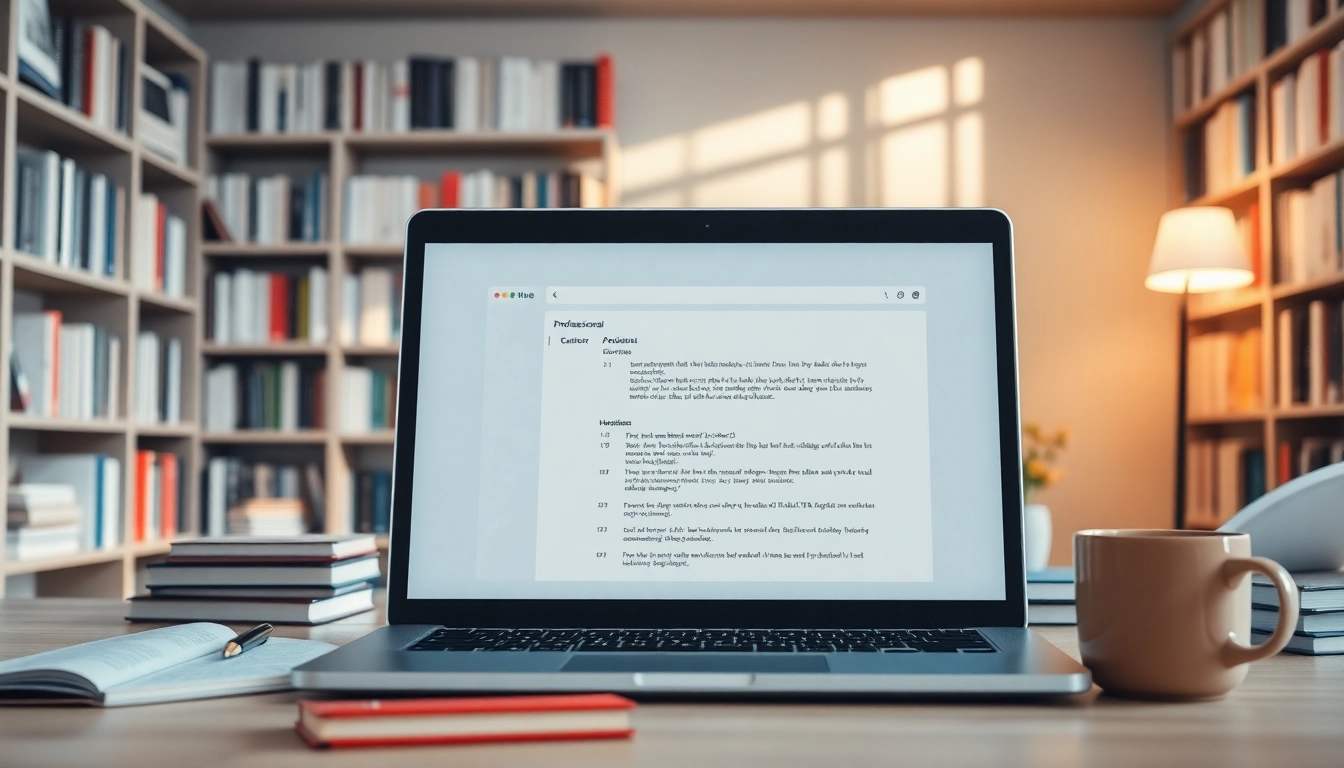
Understanding AI Summary of Papers
In an era where information is abundant and time is limited, the ability to quickly absorb critical insights from vast amounts of research is paramount. AI summary of papers emerges as a vital tool for students, researchers, and professionals alike. Through intelligent algorithms, AI summarization tools have been designed to distill extensive academic literature into concise and understandable summaries, significantly enhancing our productivity and comprehension in the academic realm.
What is AI in Research Summarization?
AI research summarization leverages natural language processing (NLP) technologies to analyze and condense research papers into shorter formats without losing essential information. These systems work by identifying key points, themes, and trends within a paper, creating a distilled version that retains the core message while eliminating superfluous details. Effectively, AI becomes an assistant—a sophisticated computational entity that significantly reduces the cognitive load of digesting scientific literature.
Benefits of Using AI for Summarizing Academic Papers
- Time Savings: Traditional reading can be time-consuming. AI summarizers can condense papers into minutes, offering quick insights without comprehensive reading.
- Enhanced Comprehension: By distilling complex language and jargon into simpler terms, these tools make research more accessible.
- Literature Reviews: Identifying the most relevant studies becomes easier, aiding in the literature review process for theses or research proposals.
- Stay Updated: With the rapid advancement of research, AI summarizers allow researchers to keep abreast of developments without sifting through an overwhelming volume of papers.
How Does AI Generate Summaries?
AI summarization typically employs two main approaches: extractive and abstractive summarization. Extractive summarization entails selecting key sentences or phrases directly from the source material to form a coherent summary. In contrast, abstractive summarization involves generating new sentences that convey the same meaning as the original text, akin to how humans summarize written work.
The algorithms underpinning these technologies rely on deep learning models trained on extensive datasets containing academic texts. Techniques such as topic modeling, sentiment analysis, and keyword recognition are employed to ensure the final summary captures essential information while maintaining readability.
Top AI Tools for Summarizing Papers
Overview of Popular AI Summarizers
Several tools stand out in the market for their efficiency and user satisfaction. Below is a brief overview of some leading AI summarizers:
- SciSummary: This tool excels in summarizing scientific articles, making it a favorite among students and researchers who need quick insights into new studies.
- Scholarcy: Known for transforming complex academic texts into interactive summary flashcards, Scholarcy also offers video summarization capabilities.
- QuillBot: An accessible text summarizer, QuillBot simplifies extensive documents into concise key points, making it ideal for general use.
- Paper Digest: This platform tracks and summarizes new papers daily, enhancing the ability to stay current with the latest research trends.
Comparative Analysis of Features
When selecting an AI summarizer, it’s crucial to evaluate various features to determine which aligns best with user needs:
Tool | Key Features | Subscription Model | Unique Selling Point |
---|---|---|---|
SciSummary | Focus on scientific articles, user-friendly interface | Free with premium plans available | Specialization in academic content |
Scholarcy | Interactive flashcards, integration with reference managers | Free and subscription options | Visual aids for easy comprehension |
QuillBot | Multi-language support, paraphrasing options | Free, premium available | User-friendly for students |
Paper Digest | Daily updates, tailored summaries by topics | Free | Real-time updates on new research |
User Reviews and Insights
Real user feedback can provide invaluable insights into the efficacy and reliability of these tools. Users often appreciate:
- The ease of use and time savings that AI summarizers provide.
- The accuracy and relevance of the generated summaries.
- Integration with existing workflows, such as reference management systems or document editors.
However, some users highlight the importance of verifying the information in AI-generated summaries, emphasizing that while AI tools are beneficial, they should complement rather than replace critical reading.
Best Practices for Using AI Summarizers
Strategies for Effectively Inputting Research Papers
To ensure that AI summarizers deliver optimal results, consider these strategies when inputting documents:
- Clear Document Structure: AI works best with well-organized papers that follow conventional structures (introduction, methodology, results, conclusion).
- Format Matters: Upload papers in formats that AI tools best understand, such as PDF or DOCX.
- Limitations of Length: Check if there are word count limitations, as some tools perform better with shorter documents.
Customizing Output for Specific Needs
Most summarization tools allow users to customize their outputs according to specific requirements:
- Select Key Topics: If your primary interest lies in particular themes, convey that to the AI to generate more relevant summaries.
- Adjust Summary Length: Depending on your needs, choose whether you need a brief overview or a more detailed examination.
Maximizing Utility in Research Workflows
Integrating AI summarizers into your research workflow can significantly enhance productivity:
- Combine with Note-Taking: Use AI summaries to complement your notes and capture critical details more effectively.
- Collaborative Platforms: Utilize tools compatible with citation management software, enabling seamless integration into your writing process.
Challenges and Limitations in AI-powered Summarization
Common Issues with AI Summarization Accuracy
While AI summarizers are proficient and generally reliable, common issues can diminish accuracy:
- Context Loss: AI may miss nuanced context or specialized terminology that could alter the significance of findings.
- Over-simplification: Summaries may oversimplify complex arguments or omit critical data leading to a skewed understanding of the paper.
Understanding the Contextual Limitations
AI’s current limitations stem from training data biases, which can lead to systematic errors. Additionally, as most tools are programmed based on algorithms, they may struggle with idiomatic expressions or cutting-edge research that hasn’t been adequately represented in their training datasets.
How to Mitigate Misinterpretations
Researchers should always cross-verify the AI-generated summaries with the original documents:
- Manual Verification: Skimming through the original paper after review helps ensure no crucial information was altered or omitted.
- Peer Discussion: Engaging fellow researchers helps provide additional insights and identifies areas where AI may have failed to capture essential details.
The Future of AI Summary of Papers
Trends in AI Technology for Academia
As AI continues to innovate, we can expect advances that will enhance research capabilities:
- Personalization: Futures where AI summarizers adapt to individual researcher preferences and prior work, resulting in tailored outputs.
- Multi-Modal Summarization: Integration of text, graphics, and audio to provide more comprehensive insights into papers, supporting different learning styles.
Potential Developments in Research Summarization Tools
New technologies may soon allow AI not only to summarize but also to synthesize conclusions from multiple papers, presenting a more holistic overview of research trends. Tools may develop capabilities to critically assess methodological rigor, providing users with even more insightful analyses.
How AI Could Transform Academic Research
The transformative nature of AI summarization technology holds promise for democratizing access to education and academic research. By lowering the barriers to understanding complex topics, AI allows for a more inclusive research community, where knowledge is more easily shared and applied globally. The future of AI in research is not just about facilitating faster information absorption; it’s about nurturing a culture of collaboration and open inquiry across disciplines.